Tweets Analysis - Keyword: @RAPIDSai
Overview
Total number of tweets analysed
40
Earliest tweet was on
2023-03-14
Latest tweet was on
2023-03-23
Tweets covering
8 days
Average age of authors' accounts
12 years
Summarization
The tweets discuss topics related to data science and machine learning, with a focus on the use of GPU acceleration and tools such as the cuSpatial library and RAPIDS.ai. Specific topics include stream-ordered memory operations, generating sparse spatial weights matrices, optimizing performance for data fusion, and using typed dict and parallelization techniques. There are also mentions of specific sessions at the GTC23 conference and discussions about the potential for further optimization in aggregation and join operations. Additionally, there are several suggestions for improving write and read times, such as changing compression methods or streamlining CUDA synchronization.
Topic Modeling
- GPU-based geospatial analytics with cuSpatial library from RAPIDSai
- Accelerating existing systems using Velox and modular composable data system building blocks
- Maximizing CUDA performance through stream-ordered memory operations
- Generating large sparse spatial weights matrix using cuGraph, ApacheArrow, CuPy_Team, numba_jit, and duckdb
- Performance optimization in DataFusion and Parquet compression overhead
Emotional Analysis
The tweets express enthusiasm and excitement about the use of @RAPIDSai for accelerating data science and machine learning workflows. They also highlight the innovative and modular building blocks that @VoltronData and other teams are using for scalable data systems. Some tweets also discuss technical challenges and solutions related to compression overhead and optimizing performance. Overall, the language in the tweets conveys a sense of passion and engagement with the field of data science and the use of cutting-edge technology.
Trend Analysis
- Use of @RAPIDSai library for GPU geospatial analytics and data science
- Modular composable data system building blocks
- High GPU utilization and scalable data science and machine learning workflows
- Optimization and performance in data processing and analysis
- Discussion and presentation of @RAPIDSai library at #GTC23
Types of Tweets
Number of Retweets
9
Percentage of total tweets
22%
Number of Original tweets
5
Percentage of total tweets
12%
Number of tweets that contain Mentions
40
Percentage of total tweets
100%
Number of tweets that were Replies
25
Percentage of total tweets
62%
Number of tweets that were Quotes
4
Percentage of total tweets
10%
Number of tweets that contain Hashtags
12
Percentage of total tweets
30%
Top 5 devices used to tweet
Source | Count |
---|---|
Twitter Web App | 29 |
Twitter for iPhone | 6 |
Twitter for Android | 5 |
What devices were used to tweet
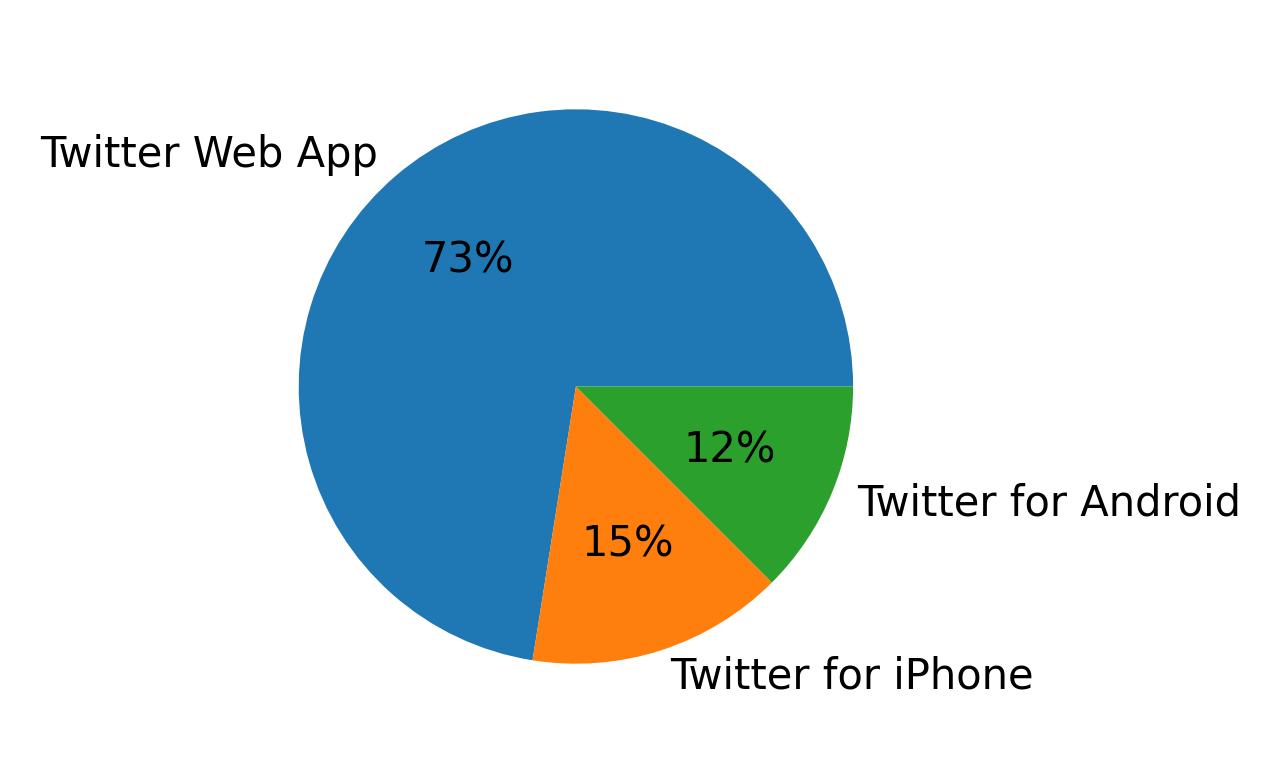
Top 10 accounts with highest followers count
Username | Name | Bio | Followers count |
---|---|---|---|
NVIDIAAIDev | NVIDIA AI Developer | All things AI for developers from @NVIDIA. Additional developer channels: @NVIDIADeveloper, @NVIDIAHPCDev, and @NVIDIAGameDev. | 37,606 |
jeffheaton | jeffheaton | YouTuber (75K+ subs), phd computer science, data scientist, and adj faculty at @WUSTL. Any opinions expressed are my own. #InsurTech #FinTech | 10,980 |
MurrayData | John Murray | Data Scientist & Visiting Professor @geodatascience. Talks about #opendata #AI #deeplearning #geospatial #GPU #HPC #kubernetes #datascience @ApacheArrow #Python | 6,513 |
TweetAtAKK | Arun Kumar | Assoc Prof at UC San Diego CSE & HDSI. HDSI Faculty Fellow. Research on data management & ML systems. Wisconsin PhD. Freethinker. Poet. Memester. Gay. He/him. | 4,576 |
datametrician | Josh Patterson | Co-founder and CEO @voltrondata. Originator of @RAPIDSai former @PIFgov (#44). Building bridges not walls. Making Data Science more efficient. | 4,450 |
harrism | Mark Harris | Software Engineer at NVIDIA. Views expressed are my own, not necessarily NVIDIA's. Software leader; developer; miller; builder; brewer; verber. | 3,941 |
ayirpelle | priya joseph | geek, entrepreneur, 'I strictly color outside the lines!', opinions r my own indeed. @ayirpelle , universal handle at this time | 3,376 |
emaxerrno | 🕺💃🤟 Alexander Gallego | Founder & CEO of @RedpandaData - A Kafka® replacement for mission critical systems. 10x Faster; Safe; API compatible. 🇨🇴 | 2,919 |
andygrove_io | Andy Grove @andygrove@fosstodon.org | @ApacheArrow PMC. Creator of DataFusion & Ballista query engines. Author of "How Query Engines Work" (https://t.co/wW1RM7dYow). GPU-Accelerating Spark @NVIDIA | 1,927 |
Bradley_Dice | Bradley Dice | GPU-powered data science at @nvidia @RAPIDSAI. PhD from @UMichPhysics @UM_MICDE, @williamjewell alum, @KCMO resident. 🖥️🧪📊🎹 (Views my own.) | 1,188 |
Top 10 accounts with highest friends count
Username | Name | Bio | Followers count |
---|---|---|---|
MurrayData | John Murray | Data Scientist & Visiting Professor @geodatascience. Talks about #opendata #AI #deeplearning #geospatial #GPU #HPC #kubernetes #datascience @ApacheArrow #Python | 7,147 |
ayirpelle | priya joseph | geek, entrepreneur, 'I strictly color outside the lines!', opinions r my own indeed. @ayirpelle , universal handle at this time | 5,000 |
Bradley_Dice | Bradley Dice | GPU-powered data science at @nvidia @RAPIDSAI. PhD from @UMichPhysics @UM_MICDE, @williamjewell alum, @KCMO resident. 🖥️🧪📊🎹 (Views my own.) | 3,152 |
shoyip | Shoichi Yip @shoyip@mastodon.bida.im | 👨🎓 busy learnin' // currently @SapienzaRoma Physics MSc // @UniTrento Physics BSc | 2,973 |
emaxerrno | 🕺💃🤟 Alexander Gallego | Founder & CEO of @RedpandaData - A Kafka® replacement for mission critical systems. 10x Faster; Safe; API compatible. 🇨🇴 | 1,660 |
keithjkraus | Keith Kraus | VP of Engineering and Co-Founder @VoltronData, @RAPIDSAI maintainer, @condaforge core. Previously @NVIDIA. My thoughts are my own. | 1,211 |
datametrician | Josh Patterson | Co-founder and CEO @voltrondata. Originator of @RAPIDSai former @PIFgov (#44). Building bridges not walls. Making Data Science more efficient. | 994 |
sardinan_guy | Roberto Panai | I was supposed to be sardinian_guy... | 993 |
andygrove_io | Andy Grove @andygrove@fosstodon.org | @ApacheArrow PMC. Creator of DataFusion & Ballista query engines. Author of "How Query Engines Work" (https://t.co/wW1RM7dYow). GPU-Accelerating Spark @NVIDIA | 587 |
miguelusque | Miguel Martínez | Deep Learning Data Scientist at NVIDIA. Challenge accepted! That will be my answer if the challenge is interesting enough. #ViewsAreMyOwn #RTsArentEndorsements | 545 |
Most active users
Username | Bio | Number of tweets |
---|---|---|
MurrayData | Data Scientist & Visiting Professor @geodatascience. Talks about #opendata #AI #deeplearning #geospatial #GPU #HPC #kubernetes #datascience @ApacheArrow #Python | 14 |
datametrician | Co-founder and CEO @voltrondata. Originator of @RAPIDSai former @PIFgov (#44). Building bridges not walls. Making Data Science more efficient. | 5 |
emaxerrno | Founder & CEO of @RedpandaData - A Kafka® replacement for mission critical systems. 10x Faster; Safe; API compatible. 🇨🇴 | 4 |
Bradley_Dice | GPU-powered data science at @nvidia @RAPIDSAI. PhD from @UMichPhysics @UM_MICDE, @williamjewell alum, @KCMO resident. 🖥️🧪📊🎹 (Views my own.) | 2 |
andygrove_io | @ApacheArrow PMC. Creator of DataFusion & Ballista query engines. Author of "How Query Engines Work" (https://t.co/wW1RM7dYow). GPU-Accelerating Spark @NVIDIA | 2 |
harrism | Software Engineer at NVIDIA. Views expressed are my own, not necessarily NVIDIA's. Software leader; developer; miller; builder; brewer; verber. | 2 |
keithjkraus | VP of Engineering and Co-Founder @VoltronData, @RAPIDSAI maintainer, @condaforge core. Previously @NVIDIA. My thoughts are my own. | 2 |
NVIDIAAIDev | All things AI for developers from @NVIDIA. Additional developer channels: @NVIDIADeveloper, @NVIDIAHPCDev, and @NVIDIAGameDev. | 1 |
TweetAtAKK | Assoc Prof at UC San Diego CSE & HDSI. HDSI Faculty Fellow. Research on data management & ML systems. Wisconsin PhD. Freethinker. Poet. Memester. Gay. He/him. | 1 |
andrewlamb1111 | Database Engineer | 1 |
Tweets per day
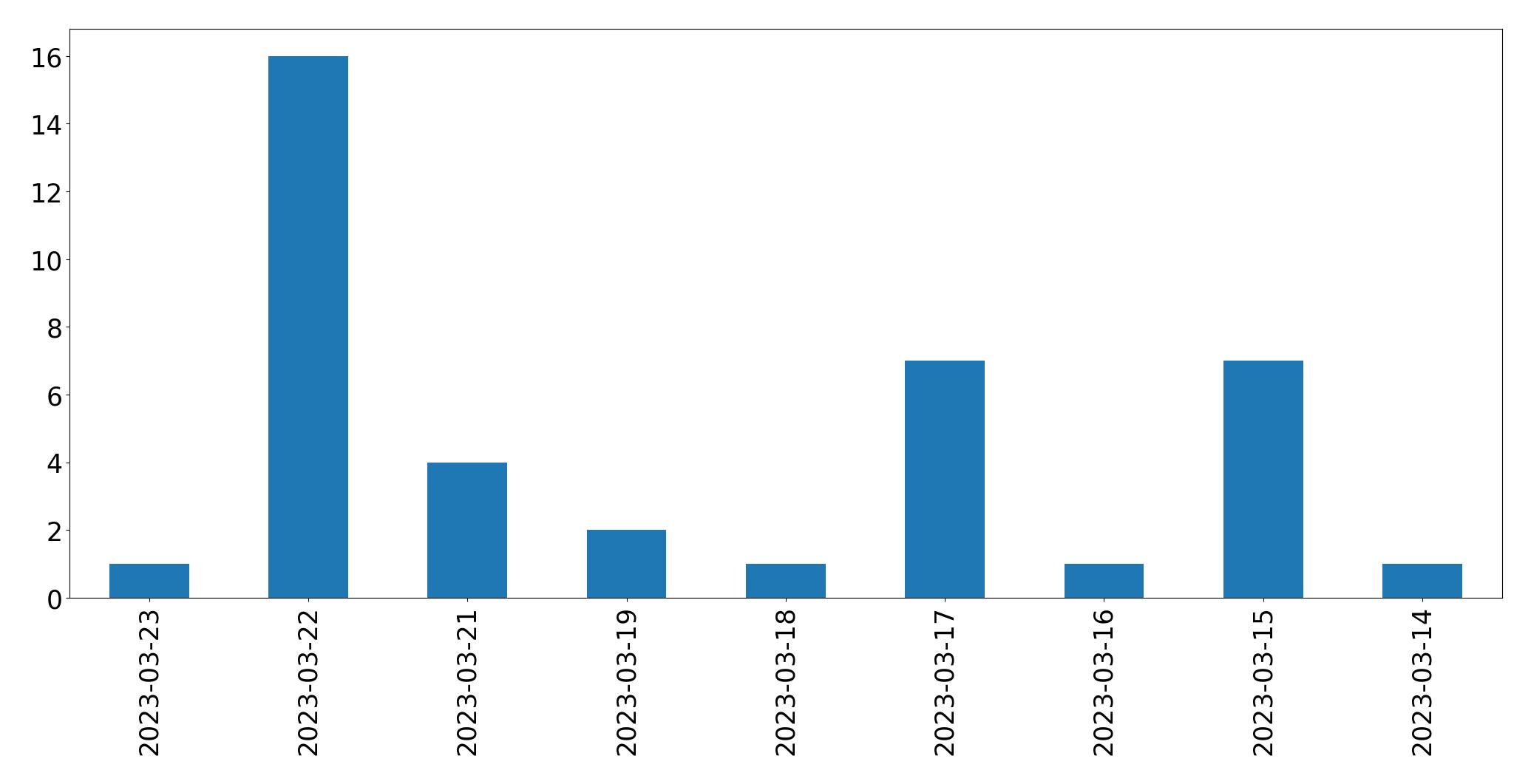
Top 10 tweets with highest Retweet count
ID | Text | Retweet count |
---|---|---|
1636024705248362496 | My colleagues Michael Wang and Thomson Comer will be presenting at #GTC23 about our work on the @RAPIDSai cuSpatial library. Join for a fascinating discussion on GPU geospatial analytics and a killer #Python demo. Don't miss it! Register now: https://t.co/V9MWbMHbPU https://t.co/1HwYoTy021 | 7 |
1638140547167559680 | Day 1 of GTC, a quick overview video of one of my favorite sessions. Accelerating Data Science with @RAPIDSai . #GTC23 @NVIDIAAI https://t.co/r1zpgeqbf7 | 4 |
1638142299040366592 | @mim_djo @DataPolars @duckdb For comparison native (non-SQL) solutions in @ApacheArrow & @RAPIDSai #cudf: cudf: 30.2s Arrow: 1m 44s https://t.co/l7TH67POPv | 1 |
1636500619891539970 | Great work from @fb_engineering @MetaOpenSource and @VoltronData’s @assignUser @raulcumplido. Velox is a vectorized executor designed to accelerate existing systems (similar to @RAPIDSai). At Voltron Data we love modular composable data system building blocks. https://t.co/VjGUtCQoUP | 1 |
1635870689818189828 | Stream-ordered memory operations are essential for maximizing CUDA performance. We achieve high GPU utilization in @RAPIDSai libraries with tools like these, enabling scalable data science and machine learning workflows. Watch Mark's talk to learn more! #GTC23 https://t.co/zwW3dvrbsd | 1 |
1638576450021343236 | @datametrician @MurrayData @mim_djo @DataPolars @duckdb @ApacheArrow @RAPIDSai It might be that `read_parquet` and `sort_values` don't synchronize the CUDA stream, whereas `to_parquet` definitely does. Maybe try adding `rmm._cuda.stream.DEFAULT_STREAM.synchronize()` at the end of the read + sort cell? | 1 |
1638500945498718209 | @MurrayData @mim_djo @DataPolars @duckdb @ApacheArrow @RAPIDSai First read is 2s (incl sort)… that’s amazing! 5x improvement from without GDS. what’s cooler is each gpu can get this perf on the same machine. Writing being 20s seems slow… not sure what’s going on. @keithjkraus might have thoughts. | 0 |
1637454380524871682 | @andygrove_io @emaxerrno @datametrician @fb_engineering @MetaOpenSource @VoltronData @assignUser @raulcumplido @RAPIDSai I think the biggest wins (factor of 2-5) remaining are in aggregation ( distinct and non distinct) and for joins of various flavors I am sure other parts can be optimized as well, but I think they are already within a factor of 2 of best in class | 0 |
1635937846551801856 | Generating a large (6tn total) sparse (12bn net) road distance spatial weights matrix for a client. Using @RAPIDSai #cuGraph, @ApacheArrow, @CuPy_Team, @numba_jit #CUDA #jitclass & typed dict, and @duckdb to generate output. 2 x E5-2697V4 CPUs, 2 x #V100 #GPU 1TB RAM. https://t.co/XxyqWgXcrr | 0 |
1635971553773879297 | GB Postcodes graph distance 1km max sparse spatial weights matrix. Points of interest, in this case postcode centroids, are matched to a road node distance table generated with @rapidsai #cuGraph, using a 750GB @numba_jit typed dict, which is parallelisable. https://t.co/UrW8DeR4j7 | 0 |
Top 10 tweets with highest Like count
ID | Text | Like count |
---|---|---|
1636024705248362496 | My colleagues Michael Wang and Thomson Comer will be presenting at #GTC23 about our work on the @RAPIDSai cuSpatial library. Join for a fascinating discussion on GPU geospatial analytics and a killer #Python demo. Don't miss it! Register now: https://t.co/V9MWbMHbPU https://t.co/1HwYoTy021 | 18 |
1636500619891539970 | Great work from @fb_engineering @MetaOpenSource and @VoltronData’s @assignUser @raulcumplido. Velox is a vectorized executor designed to accelerate existing systems (similar to @RAPIDSai). At Voltron Data we love modular composable data system building blocks. https://t.co/VjGUtCQoUP | 18 |
1638140547167559680 | Day 1 of GTC, a quick overview video of one of my favorite sessions. Accelerating Data Science with @RAPIDSai . #GTC23 @NVIDIAAI https://t.co/r1zpgeqbf7 | 16 |
1635870689818189828 | Stream-ordered memory operations are essential for maximizing CUDA performance. We achieve high GPU utilization in @RAPIDSai libraries with tools like these, enabling scalable data science and machine learning workflows. Watch Mark's talk to learn more! #GTC23 https://t.co/zwW3dvrbsd | 7 |
1635937846551801856 | Generating a large (6tn total) sparse (12bn net) road distance spatial weights matrix for a client. Using @RAPIDSai #cuGraph, @ApacheArrow, @CuPy_Team, @numba_jit #CUDA #jitclass & typed dict, and @duckdb to generate output. 2 x E5-2697V4 CPUs, 2 x #V100 #GPU 1TB RAM. https://t.co/XxyqWgXcrr | 6 |
1638473809694019585 | @datametrician @mim_djo @DataPolars @duckdb @ApacheArrow @RAPIDSai Interesting results, Josh, with GDS now working. It's cut the read time in cudf from 10 seconds to 2, but the write (same device) has hardly changed. Parquet compression overhead? https://t.co/w41rGbeFsq | 5 |
1637454380524871682 | @andygrove_io @emaxerrno @datametrician @fb_engineering @MetaOpenSource @VoltronData @assignUser @raulcumplido @RAPIDSai I think the biggest wins (factor of 2-5) remaining are in aggregation ( distinct and non distinct) and for joins of various flavors I am sure other parts can be optimized as well, but I think they are already within a factor of 2 of best in class | 4 |
1638147168560136194 | I'm looking forward to @zstats session on @rapidsai 'Accelerate Data Science in Python with RAPIDS, with Q&A from EMEA Region [S51281a]' at #GTC23 in a few minutes. Link to talk: https://t.co/apooqjUuuD | 3 |
1635971553773879297 | GB Postcodes graph distance 1km max sparse spatial weights matrix. Points of interest, in this case postcode centroids, are matched to a road node distance table generated with @rapidsai #cuGraph, using a 750GB @numba_jit typed dict, which is parallelisable. https://t.co/UrW8DeR4j7 | 3 |
1636766323161325568 | @datametrician @emaxerrno @fb_engineering @MetaOpenSource @VoltronData @assignUser @raulcumplido @RAPIDSai I agree with this assessment. There is a lot more work to do in DataFusion to get state-of-the-art performance, but given the traction of the library, I expect contributors will become increasingly motivated to put in the work to get there. | 3 |
Top 3 Languages Used In Tweets
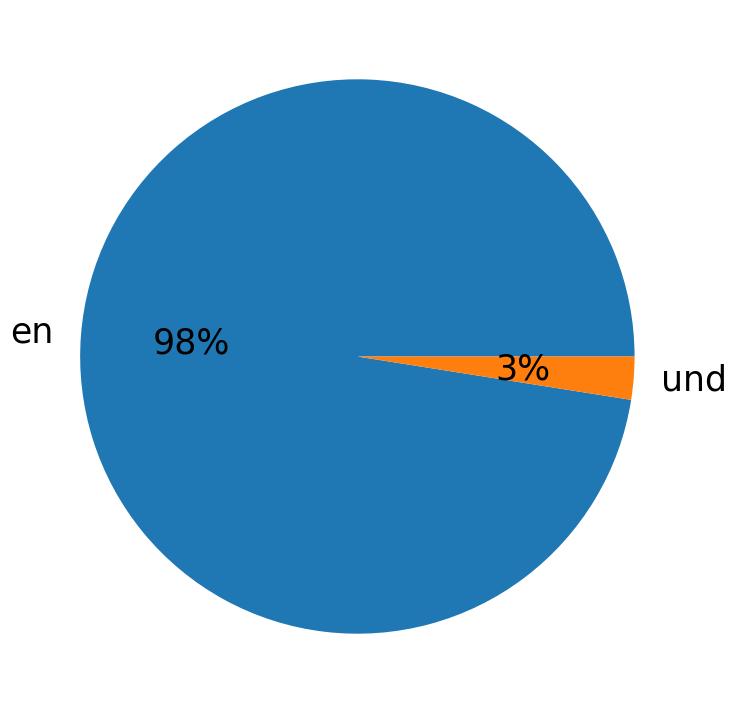
Top 10 Hashtags used
Hashtag | Count |
---|---|
#gtc23 | 7 |
#cudf | 2 |
#python | 2 |
#cugraph | 2 |
#cuda | 1 |
#jitclass | 1 |
#v100 | 1 |
#gpu | 1 |
#rapids | 1 |
Top 10 Hashtags Used In Tweets
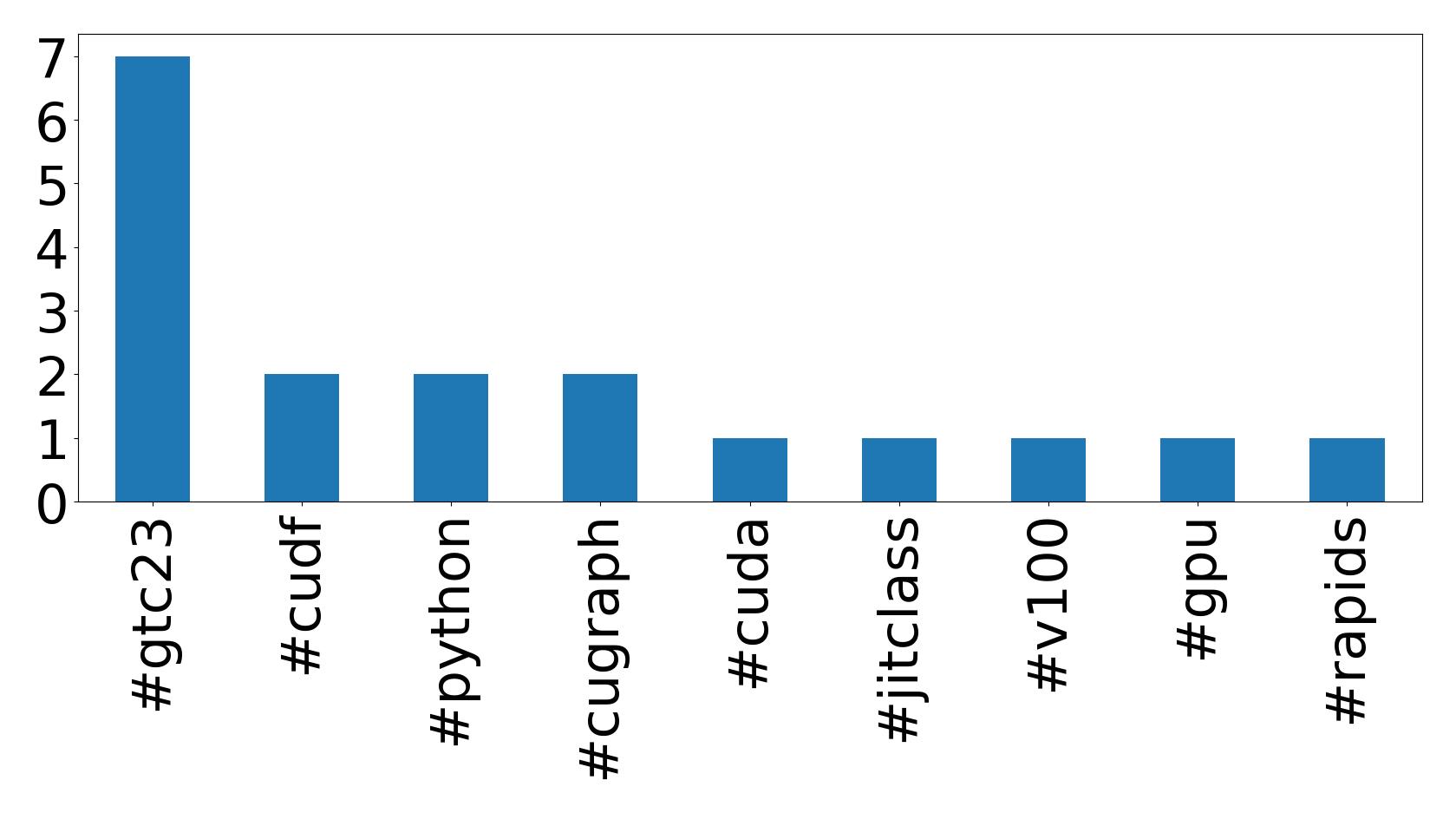
Top 10 mentions
Mention | Count |
---|---|
@rapidsai | 40 |
@datametrician | 20 |
@duckdb | 18 |
@apachearrow | 18 |
@mim_djo | 17 |
@datapolars | 17 |
@fb_engineering | 10 |
@metaopensource | 10 |
@voltrondata | 10 |
@assignuser | 10 |
Top 10 mentions
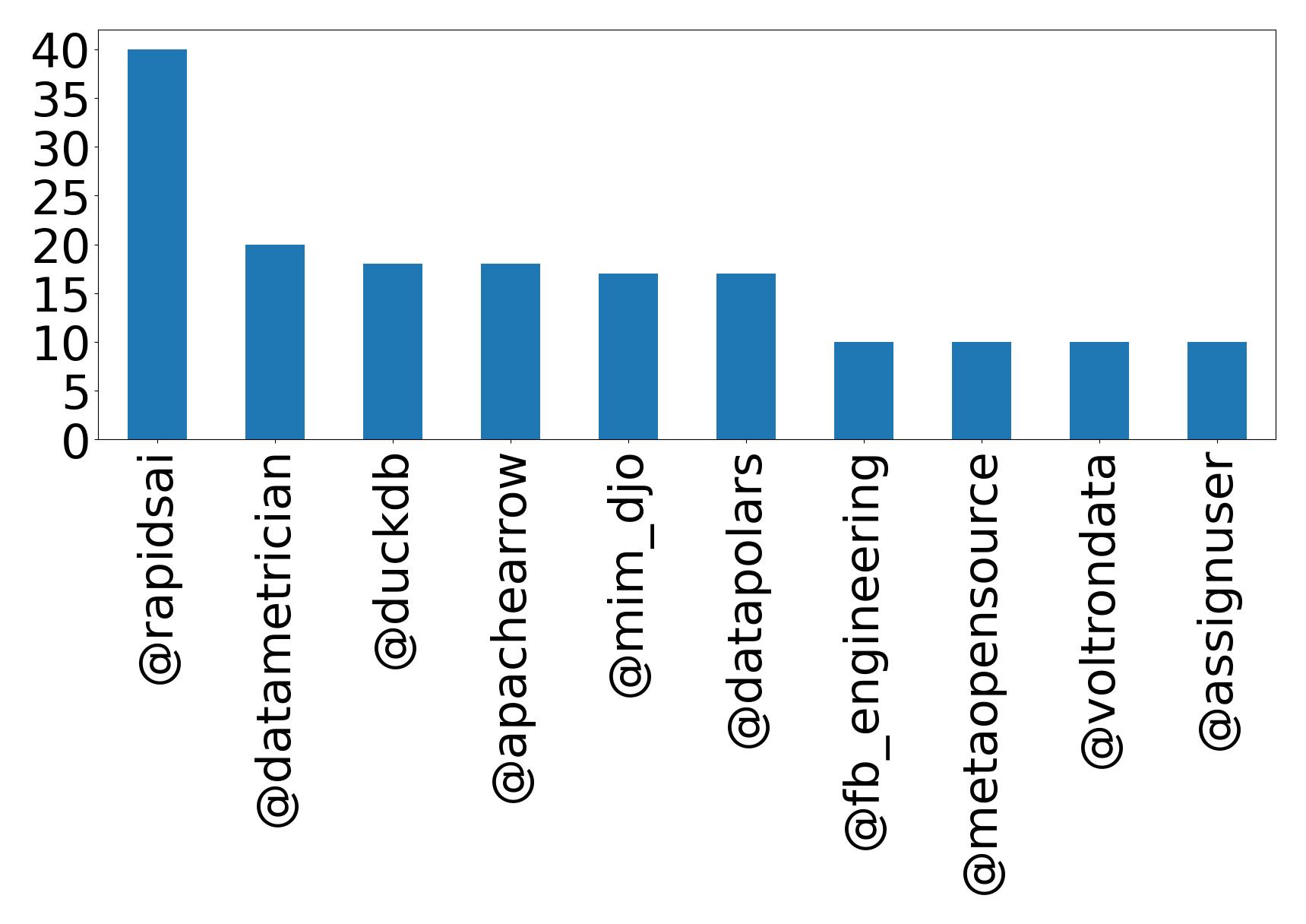
Wordcloud of Tweets
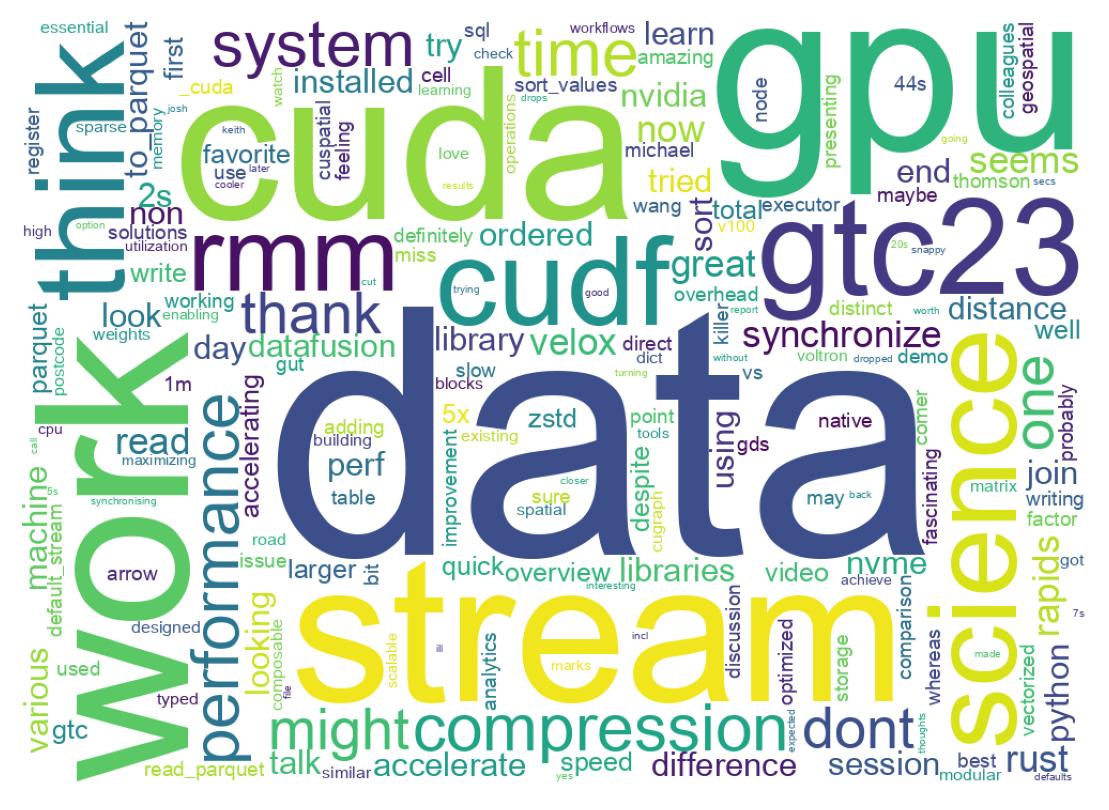
Emojis
Average number of emojis used per tweet
5
Emojis used in tweets
Emoji | Count | Emoji Text |
---|---|---|
👇 | 1 | backhand_index_pointing_down |
👀 | 1 | eyes |
Emojis groups
Emoji Group | Count |
---|---|
People & Body | 2 |